일 | 월 | 화 | 수 | 목 | 금 | 토 |
---|---|---|---|---|---|---|
1 | 2 | 3 | 4 | 5 | ||
6 | 7 | 8 | 9 | 10 | 11 | 12 |
13 | 14 | 15 | 16 | 17 | 18 | 19 |
20 | 21 | 22 | 23 | 24 | 25 | 26 |
27 | 28 | 29 | 30 |
- Value too long for column
- preemption #
- JanusWebRTC
- tolerated
- 코루틴 빌더
- vfr video
- 티스토리챌린지
- 코루틴 컨텍스트
- table not found
- JanusWebRTCGateway
- 깡돼후
- mp4fpsmod
- 헥사고날아키텍처 #육각형아키텍처 #유스케이스
- 개성국밥
- PersistenceContext
- 자원부족
- terminal
- 겨울 부산
- JanusGateway
- JanusWebRTCServer
- 달인막창
- kotlin
- VARCHAR (1)
- python
- 오블완
- Spring Batch
- k8s #kubernetes #쿠버네티스
- taint
- pytest
- PytestPluginManager
너와 나의 스토리
time series data 다루기 - kaggle 실습 / pandas 함수 정리 본문
time series data 다루기 - kaggle 실습 / pandas 함수 정리
노는게제일좋아! 2019. 7. 22. 15:40kaggle(https://www.kaggle.com/szrlee/stock-time-series-20050101-to-20171231#CAT_2006-01-01_to_2018-01-01.csv)에 있는 데이터로 실습함
* Kernels을 참조
할 것
1. google과 cat 데이터를 load
2. 한 달 단위로 나눠서 learning 할 것
3. 미래의 결과 예측
1. Introduction to date and time
1.1 import
.
.
import numpy as np
import pandas as pd
import matplotlib.pyplot as plt
.
.
1.2 데이터 로드
jupyter notebook에 현재 문서가 있는 곳에 input파일을 만들고 그 곳에 csv파일을 load한다.
google = pd.read_csv('./input/GOOGL_2006-01-01_to_2018-01-01.csv', index_col='Date', parse_dates=['Date'])
google.head()
index_col='Date' -> 날짜를 인덱스로 설정하겠다
parse_date: 인덱스 열이나 복수 열의 날짜를 파싱할지 여부
* 만약 데이터에 NaN 값이 존재한다면
google=google.iloc[1: ] # 1행~
google=google.fillna(method='ffill') # null 값들이 마지막 값으로 채워짐
* iloc / loc
.iloc[[행],[열]] : 행, 열을 지정해서 데이터 얻음
ex) .iloc[: , :3] : 모든 행(:) + [0,1,2]열
* fillna
.fillna(0): NaN값들이 모두 0으로 채워짐
.fillna(data,limit=2): 연속된 NaN 값 중 몇번째 값(2)까지 변경할지 지정
.fillna(method='ffill'): NaN 값이 그 앞 데이터로부터 채워짐
.fillna(method-'bfill'): NaN 값이 그 뒤 데이터로부터 채워짐
1.3 데이터 셋 시각화 하기
google['2008':'2010'].plot(subplots=True, figsize(10,12))
plt.title('Google stock attributes from 2008 to 2010')
plt.savefig('stocks.png')
plt.show()
figsize(): 그래프 가로 세로 비율 설정
* Matplotlib - 데이터를 차트나 플롯으로 그려주는 라이브러리 패키지
1.4 Timestamps and Periods
timestamp = pd.Timestamp(2017,1,1,12) # 해당 시점이 저장됨
timestamp # 출력: Timestamp('2017-01-01 12:00:00')
period = pd.Period('2017-01-01') -> 2017.01.01 하루라는 기간을 저장
period # 출력: Period('2017-01-01', 'D')
#해당 period에 timestamp가 존재하는지 확인해보기
period.start_time < timestamp < period.end_time # 출력: True
#period를 timestamp로 바꾸기
new_timestamp = period.to_timestamp(freq='H', how ='start')
new_timestamp # 출력: Timestamp('2017-01-01 00:00:00')
freq 인수로 특정 기간 선택
- s: 초
- T: 분
- H: 시간
- D: 일(day)
- B: 주말이 아닌 평일
- W: 주(일요일)
- W-MON: 주(월요일)
- M: 각 달(month)의 마지막 날
- MS: 각 달의 첫날
- BM: 주말이 아닌 평일 중에서 각 달의 마지막 날
- BMS: 주말이 아닌 평일 중에서 각 달의 첫날
- WOM-2THU: 각 달의 두번째 목요일
- Q-JAN: 각 분기의 첫달의 마지막 날
- Q-DEC: 각 분기의 마지막 달의 마지막 날
1.5 date_range 사용
date_range(): fixed frequency datetimeindex를 리턴함. 일정 기간 내의 인덱스를 생성한다.
dr1= pd.date_range(start='1/1/18', end='1/9/18')
dr1
'''
출력
DatetimeIndex(['2018-01-01', '2018-01-02', '2018-01-03', '2018-01-04',
'2018-01-05', '2018-01-06', '2018-01-07', '2018-01-08',
'2018-01-09'],
dtype='datetime64[ns]', freq='D')
'''
dr2 = pd.date_range(start='1/1/18', end='1/1/19', freq='M') #freq='M': 각 달의 마지막 출력
dr2
...
출력
DatetimeIndex(['2018-01-31', '2018-02-28', '2018-03-31', '2018-04-30',
'2018-05-31', '2018-06-30', '2018-07-31', '2018-08-31',
'2018-09-30', '2018-10-31', '2018-11-30', '2018-12-31'],
dtype='datetime64[ns]', freq='M')
...
dr3 = pd.date_range(end='1/4/2014', periods=8)
dr3
...
출력
DatetimeIndex(['2013-12-28', '2013-12-29', '2013-12-30', '2013-12-31',
'2014-01-01', '2014-01-02', '2014-01-03', '2014-01-04'],
dtype='datetime64[ns]', freq='D')
...
dr4 = pd.date_range(start='2013-04-24', end='2014-11-27', periods=3)
dr4
...
출력
atetimeIndex(['2013-04-24', '2014-02-09', '2014-11-27'], dtype='datetime64[ns]', freq=None)
...
1.6 to_datetime 사용
to_datetime(): 인수를 datetime으로 변환. DataFrame은 datetime series로 변환됨
df = pd.DataFrame({'year': [2015, 2016], 'month': [2, 3], 'day': [4, 5]})
df
출력:
year | month | day | |
0 | 2015 | 2 | 4 |
1 | 2016 | 3 | 5 |
df=pd.to_datetime('01-01-2017')
df
'''
출력
Timestamp('2017-01-01 00:00:00')
...
1.7 Resampling
Downsampling: time series가 high frequency에서 low frequency로 resampled됨.(Weekly to monthly frequency)
기존 데이터를 집계하는 작업
#resampling(downsampling)하기 전에 Shape
pressure.shape #출력: (45252, 36)
#평균을 사용하여 hourly에서 3 day frequency aggregated를 downsample
pressure=pressure.resample('3D').mean()
pressure.head() # 처음 5개 row 출력
#resampling(downsampling) 후에 Shape
pressure.shape #출력: (629, 36)
Unsampling: time series은 low frequency에서 high frequency로 resampled된다(Monthly to daily frequency).
누락된 데이터를 채우는 작업
#3 day frequency에서 daily frequency로 upsample
pressure = pressure.resample('D').pad()
#resampling(upsampling) 후에 Shape
pressure.shape #출력: (1885, 36)
* NumPy 배열의 구조는 "Shape"으로 표현된다. Shape은 배열의 구조를 파이썬 튜플 자료형을 이용하여 정의한다.
ex) Shape이 (28,28,3)이면 3차원 배열
1.8 Shift
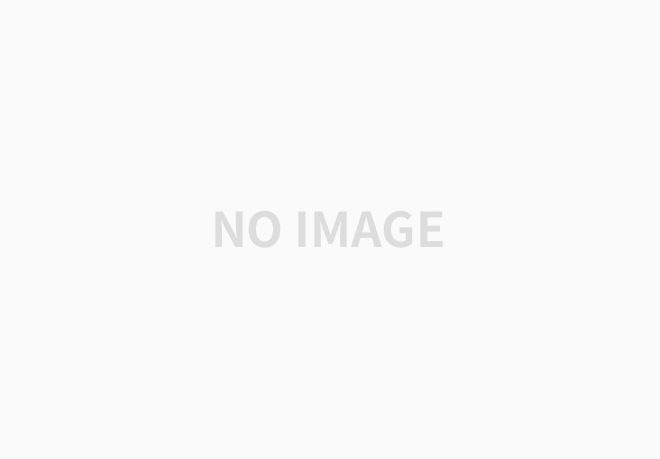
양의 방향으로 2개의 periods로 index 축을 이동 (위에서 아래로 2개만큼 값들을 내림)
axis=0은 default
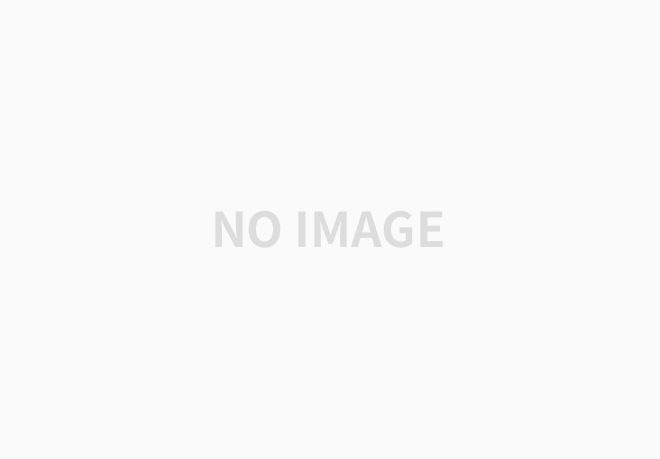
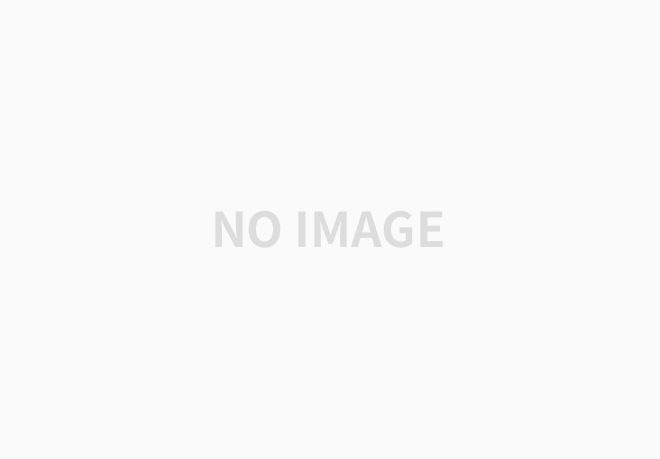
* df/s == df.div(s,axis=0)
2. Finance and Statistics
2.1 Percent change
google['Change'] = google.High.div(google.High.shift())
google['Change'].plot(figsize=(20,8))
2.2 Absolute change in successive rows
google.High.diff().plot(figsize=(20,6))
2.3 두 개 이상의 time series 비교하기
갹 time series들을 normalizing해서 비교할 것
모든 time series element를 첫 번째 element로 나누면 됨
그럼 두 series가 같은 지점에서 시작되어 쉽게 비교할 수 있음
#Plotting before normalization
google.High.plot()
cat.High.plot()
plt.legend(['Google','Cat'])
plt.show()
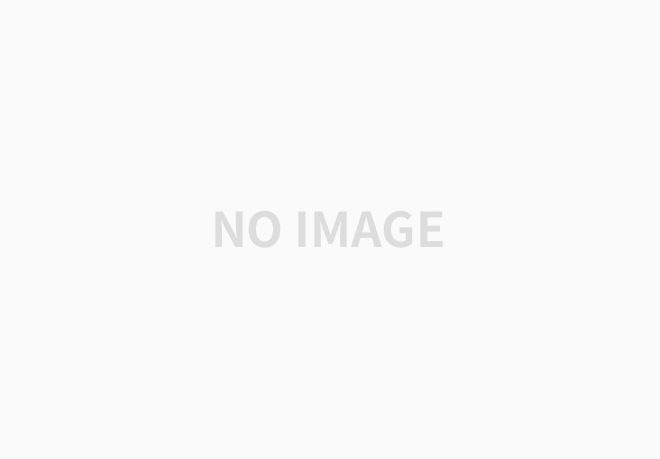
#Normalizing and comparison
#Both stocks start from 100
normalized_google = google.High.div(google.High.iloc[0]).mul(100)
normalized_cat=cat.High.div(cat.High.iloc[0]).mul(100)
normalized_google.plot()
normalized_cat.plot()
plt.legend(['Google','Cat'])
plt.show()
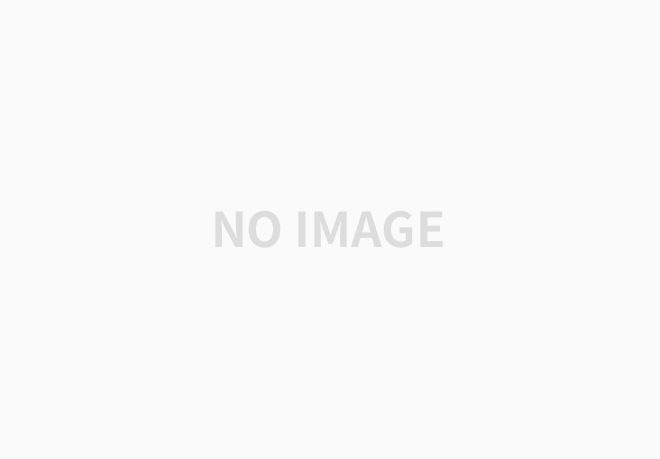
2.4 Window functions
: 행과 행간의 관계를 쉽게 정의 하기 위해 만든 함수
Rolling: Same size and sliding(동일한 사이즈만큼 옆으로 이동하면서 연산)
일정 범위에서 동일한 연산을 할 때 유용
ex) 사이즈가 2라고, 평균 내는 연산을 한다면
(1,2) 평균 내고 (2,3) 평균 (3,4) 이런식으로 진행됨
# Rolling window functions
rolling_google = google.High.rolling('90D').mean() #순차적으로 90 day씩 평균낸다
google.High.plot()
rolling_google.plot()
plt.legend(['High','Rolling Mean'])
# Plotting a rolling mean of 90 day window with original High attribute of google stocks
plt.show()
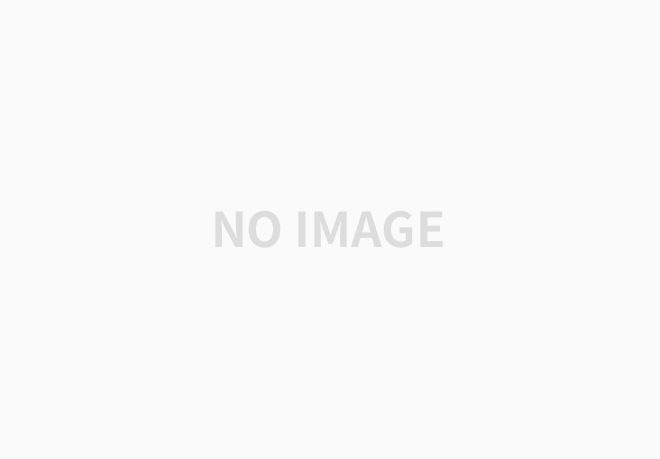
Expanding: Contains all prior values(사이즈 키워가며 연산)
ex) 평균 내기
(1,2) 평균 (1,2,3) 평균 (1,2,3,4) 평균 ....
#Expanding window functions
cat_mean = cat.High.expanding().mean()
cat_std=cat.High.expanding().std()
cat.High.plot()
cat_mean.plot()
cat_std.plot()
plt.legend(['High','Expanding Mean','Expanding Standard Deviation'])
plt.show()
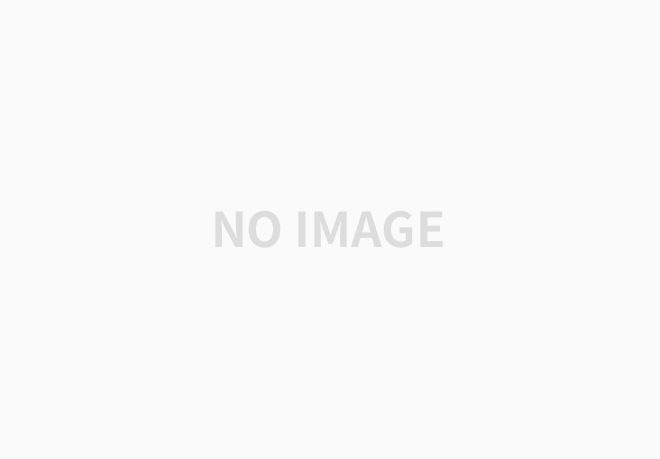
2.5 Autocorrelation and Partial Atocorrelation
Autocorrelation: autocorrelation function은 서로 다른 lags에서 series가 어떻게 상호 연관되는지 측정한다.
lag K에서, K 간격을 두고 있는 series 값과 현재 t 사이의 상관 관계
즉, 시점 t와 시점 t-k 둘 사이의 상관관계
Partial Autocorrelation: partial autocorrelation function은 과거 lag에 대한 series regression으로 해석할 수 있다.
표준 선형 회귀 분석과 동일한 방식으로 해석될 수 있다.
즉, 다른 용어는 일정하게 유지하면서도 특정 지연의 변화 기여를 의미한다.
lag K에서, K 간격을 두고 있는 series 값과 현재 t 시점 사이의 모든 상관 관계
즉, 시점 t와 시점 t-k 둘 사이의 모든 시점들과의 상관관계
3 Time series decompostion and Random walks
3.1 Trends, seasonlaity and noise
Trend: 일관된 time series의 상승 하강 기울기
Seasonality: 싸인 함수처럼 time series의 주기적인 패턴
Noise: 특이치 또는 누락된 값
# Now, for decomposition...
rcParams['figure.figsize'] = 11, 9
decomposed_google_volume = sm.tsa.seasonal_decompose(google["High"],freq=360) # The frequncy is annual
figure = decomposed_google_volume.plot()
plt.show()
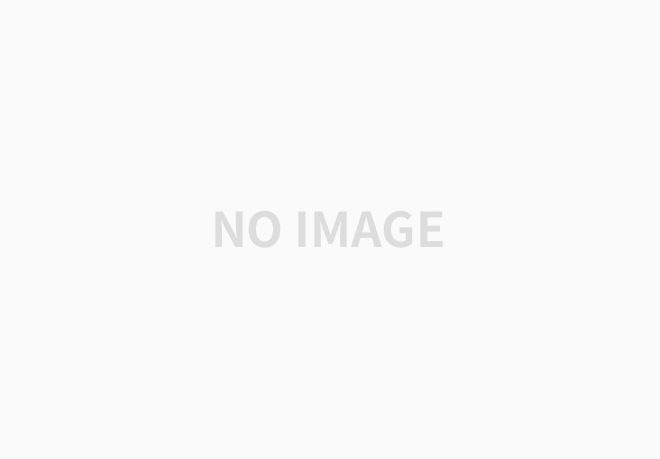
trend 또는 seasonality를 지닌 time series는 non-stationary하다.
이들은 서로 다른 시간대의 time series 값에 영향을 미치기 때문이다.
3.2 White noise
time series가 white noise인 경우, 그것은 무작위 숫자의 순서여서 예측할 수 없다.
series의 예측 오류가 white noise가 아니라면 예측 모델에 개선이 이루어질 수 있음을 시사한다.
time series는 변수가 독립적이고 평균 0으로 동일하게 분포된 경우 white noise이다.
이는 모든 변수가 동일한 분산을 가지며 각 값은 series의 다른 모든 값과 0의 상관관계를 갖는다는 것을 의미한다.
white noise는 stationary하다.
series의 변수가 가우스 분포에서 도출된 경우 serires를 Gaussian white noise라고 한다.
3.3 Random Walk
Random walk는 확률적 또는 무작위 과정으로 알려진 수학적 개념으로, 정수와 같은 일부 수학적인 공간에 대한 일련의 무작위 스텝으로 구성된 경로를 설명한다.
일반적으로 주식 얘기하자면, 오늘의 가격=어제의 가격+ noise
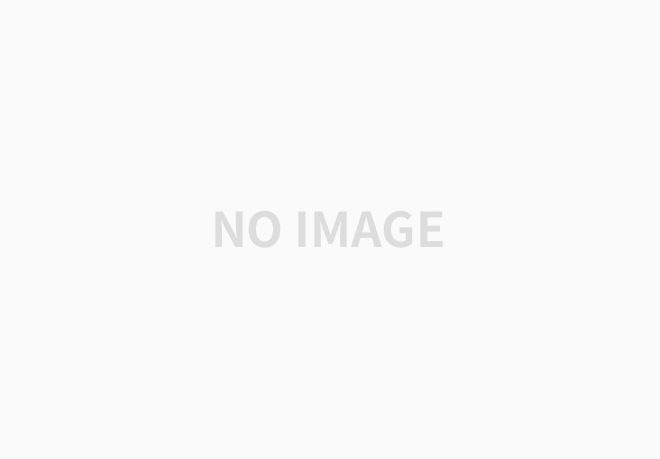
Random walk는 noise가 랜덤하기 때문에 잘 예측되지 않는다.
Random walk는 시간에 따른 편차의 평균이 0이지만 분산은 시간에 비례하여 증가하게 된다. 따라서, 앞뒤로 움직일 확률이 동일하다고 해도 시간이 흐름에 따라 평균에서 점차 벗어나는 경향을 보인다.
3.4 Stationarity
Stationarity time series는 시간이 지남에 따라 모두 일정한 평균, 분산, 상관관계 등과 같은 통계적 특성 중 하나이다.
Strong stationarity: 시간 이동시 무조건적으로 joint probability distribution가 변하지 않는 확률적 과정이다.
즉, 평균이나 분산 같은 파라미터는 시간이 지나도 변하지 않는다
Weak stationarity: 평균, 분산, 상관관계가 시간에 따라 항상 동일한 과정
시간에 따라 달라지는 non-stationary series는 time series를 모델링할 때 고려해야 할 파라미터가 너무 많기 때문에 stationarity가 중요하다. diff() method는 non-stationary series를 stationary series로 변환시켜 준다.
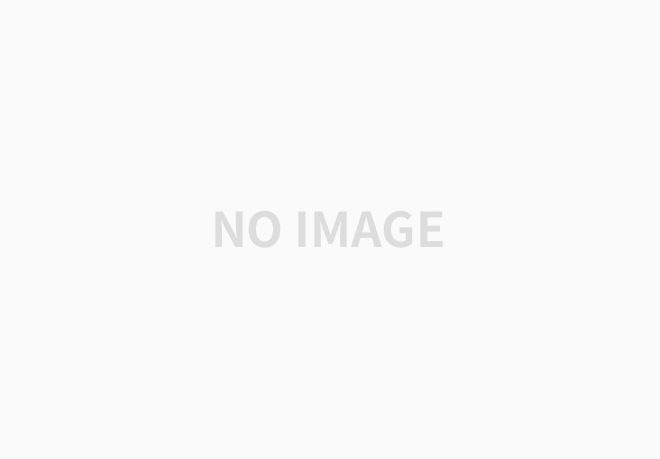
4. Modelling using statstools
4.1 AR models
AR(autoregressive) 모델은 random process의 유형을 나타내는 것이다. 따라서 자연, 경제 등에서 특정 시간 변동 프로세스를 설명하는데 사용된다. AR 모델은 출력 변수가 이전의 값과 확률적 용어(완벽하게 예측 불가능한 용어)에 따라 선형적으로 의존한다고 명시한다. 따라서 모델은 확률적 차이 방정식의 형태를 띤다.
AR models: white noise의 현재 값과 자기 자신의 과거값의 선형 가중합으로 이루어진 정상 확률 모형
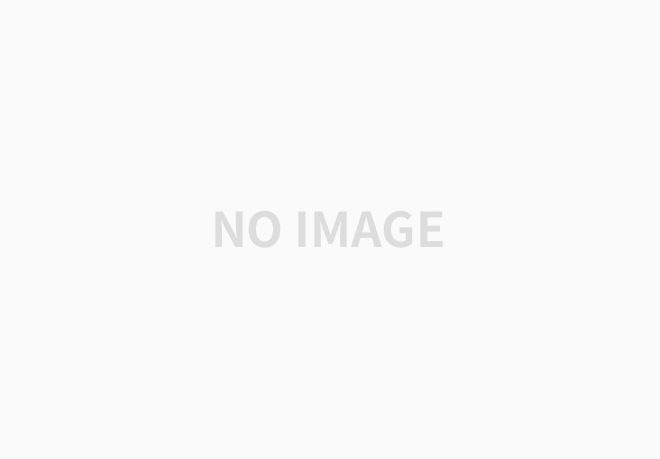
εt: white noise
<-
* 참고하기 - https://datascienceschool.net/view-notebook/e880ecd416554dbf84db6a3e8f426b99/
Simulating AR(1) model
# AR(1) MA(1) model:AR parameter = +0.9
rcParams['figure.figsize'] = 16, 12
plt.subplot(4,1,1)
ar1 = np.array([1, -0.9]) # We choose -0.9 as AR parameter is +0.9
ma1 = np.array([1])
AR1 = ArmaProcess(ar1, ma1)
sim1 = AR1.generate_sample(nsample=1000)
plt.title('AR(1) model: AR parameter = +0.9')
plt.plot(sim1)
# We will take care of MA model later
# AR(1) MA(1) AR parameter = -0.9
plt.subplot(4,1,2)
ar2 = np.array([1, 0.9]) # We choose +0.9 as AR parameter is -0.9
ma2 = np.array([1])
AR2 = ArmaProcess(ar2, ma2)
sim2 = AR2.generate_sample(nsample=1000)
plt.title('AR(1) model: AR parameter = -0.9')
plt.plot(sim2)
# AR(2) MA(1) AR parameter = 0.9
plt.subplot(4,1,3)
ar3 = np.array([2, -0.9]) # We choose -0.9 as AR parameter is +0.9
ma3 = np.array([1])
AR3 = ArmaProcess(ar3, ma3)
sim3 = AR3.generate_sample(nsample=1000)
plt.title('AR(2) model: AR parameter = +0.9')
plt.plot(sim3)
# AR(2) MA(1) AR parameter = -0.9
plt.subplot(4,1,4)
ar4 = np.array([2, 0.9]) # We choose +0.9 as AR parameter is -0.9
ma4 = np.array([1])
AR4 = ArmaProcess(ar4, ma4)
sim4 = AR4.generate_sample(nsample=1000)
plt.title('AR(2) model: AR parameter = -0.9')
plt.plot(sim4)
plt.show()
Forecasting a simulated model
model = ARM(sim1, order=(1,0))
result= model.fit()
ϕ는 약 0.9이며, 이것이 우리가 처음 시뮬레이션한 모델에서 AR 파라미터로 선택한 것이다.
Predicting the models
result.plot_predict(start=900,end=1010)
plt.show()
rmse = math.sqrt(mean_squared_error(sim1[900:1011], result.predict(start=900,end=999)))
print("The root mean squared error is {}.".format(rmse))
#출력: The root mean squared error is 1.0408054544358292.
4.2 MA models
MA(moving-average) model은 일변량 time series 모델링을 위한 공통 접근방식이다.
MA 모델은 출력 변수가 확률적 term의 현재 및 다양한 과거 값에 따라 선형적으로 좌우됨을 명시한다.
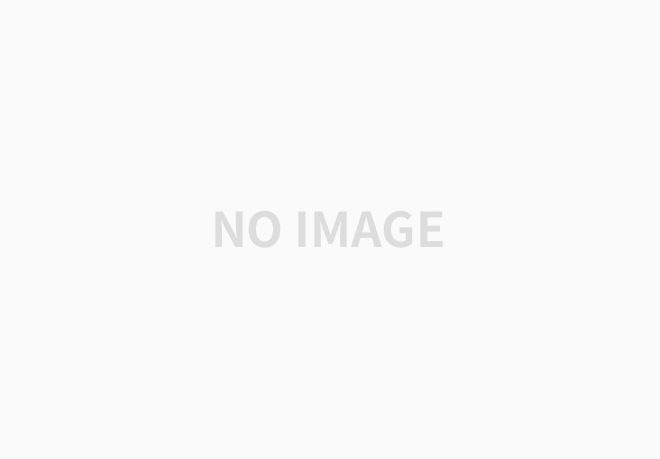
=> Today's returns = mean + today's noise + yesterday's noise
Simulating MA(1) model
rcParams['figure.figsize'] = 16, 6
ar1 = np.array([1])
ma1 = np.array([1, -0.5])
MA1 = ArmaProcess(ar1, ma1)
sim1 = MA1.generate_sample(nsample=1000)
plt.plot(sim1)
Forecasting the simulated MA model
model = ARMA(sim1, order=(0,1))
result = model.fit()
Prediction using MA models
# Forecasting and predicting montreal humidity
model = ARMA(humidity["Montreal"].diff().iloc[1:].values, order=(0,3))
result = model.fit()
print(result.summary())
print("μ={} ,θ={}".format(result.params[0],result.params[1]))
result.plot_predict(start=1000, end=1100)
plt.show()
4.3 ARMA models
ARMA(Autoregressive moving average) 모델은 두 개의 다항식(AR, MA)의 관점에서 (weakly) stationary stochastic process에 대한 모사적인 설명을 제공. AR과 MA 모델의 융합이다.
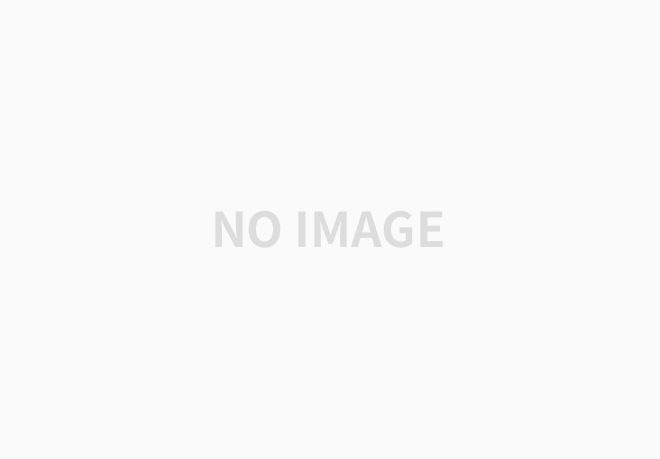
=> Today's return = mean + Yesterday's return + noise + yesterday's noise.
Prediction using ARMA models
'Data Analysis > Machine learning' 카테고리의 다른 글
RNN & LSTM & GRU 완벽 정리 (0) | 2019.07.25 |
---|---|
RNN의 변형 - LSTM(Long Short Term Memory) (0) | 2019.07.24 |
[Machine Learning - google developers] 손실 줄이기 (0) | 2019.07.19 |
[Machine Learning - google developers] ML로 전환하기 - 선형 회귀 / 학습 및 손실 (0) | 2019.07.19 |
GBM(Gradient Boosting) (0) | 2019.07.18 |