일 | 월 | 화 | 수 | 목 | 금 | 토 |
---|---|---|---|---|---|---|
1 | 2 | 3 | 4 | 5 | ||
6 | 7 | 8 | 9 | 10 | 11 | 12 |
13 | 14 | 15 | 16 | 17 | 18 | 19 |
20 | 21 | 22 | 23 | 24 | 25 | 26 |
27 | 28 | 29 | 30 |
- pytest
- 겨울 부산
- mp4fpsmod
- 오블완
- 코루틴 빌더
- JanusGateway
- vfr video
- JanusWebRTC
- preemption #
- tolerated
- 코루틴 컨텍스트
- table not found
- k8s #kubernetes #쿠버네티스
- JanusWebRTCGateway
- JanusWebRTCServer
- 깡돼후
- VARCHAR (1)
- kotlin
- PersistenceContext
- 개성국밥
- terminal
- Value too long for column
- 자원부족
- 헥사고날아키텍처 #육각형아키텍처 #유스케이스
- python
- PytestPluginManager
- 티스토리챌린지
- 달인막창
- Spring Batch
- taint
목록Data Analysis (88)
너와 나의 스토리
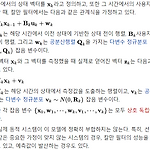
● SGD(Stochastic gradient descent) 배치 사이즈가 1(즉, 하나의 예)인 미니배치에서 gradient를 계산하는 것 gradient를 평가하기 위해 무작위로 선택된 샘플을 사용 반복이 충분하면 SGD는 효과 있지만 noise가 심함 first-order gradient 정보만 이용 second-order gradient 기반 기술보다 수렴이 느리고 조건이 좋지 않은 문제에서 성능이 저하된다 이를 완화시키기 위해 second-order gradient를 고려한다 수렴률(convergence rate)이 빠르고 conditioned problem에 대해 더 강력하다 Hessian Matrix라는 2차 편미분 행렬을 계산한 후 역행렬을 구해야 한다. SGD algorithm 기반의 ..
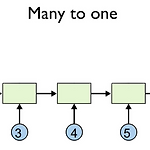
모두를 위한 딥러닝 ML lab12-6: RNN with Time Series Data 강의 정리 & 코드 전체 코드: https://github.com/hunkim/DeepLearningZeroToAll/blob/master/lab-12-5-rnn_stock_prediction.py RNN으로 time series data인 주식 시장을 예측해보자 Many to one Dataset input dimension = 5 [open, high, low, volume, close] sequence = 7 [7일 동안의 데이터를 토대로 수행] output dimension = 1 [8일 째만 예측] 1. import import tensorflow as tf import numpy as np import ma..
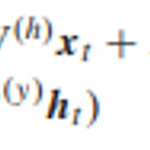
"Efficient Online Learning Algorithms Based on LSTM Neural Networks" Tolga Ergen and Suleyman Serdar Kozat, Senior Member, IEEE Abstract 우리는 nonlinear regression을 조사하고, LSTM network 기반의 novel regression structures를 소개한다. 이 구조를 설명하기 위해 효과적인 online training 방법을 제공한다. novel LSTM 기반 구조를 훈련시키기 위해서 우리는 기본 아키텍처를 주 공간 형태로 배치하고 매우 효율적인 PF(particle filtering) 기반 업데이트를 도입한다. 우리는 또한 확률적 경사 강하(stochastic gra..
NN(Neural Network) 설명 Deep learning은 ANN(Artificial Neural Network)를 기초로 하고 있다. 퍼셉트론에서 특정 임계치를 넘어야 신경이 전달되는 것을 Sigmoid function을 이용하여, weight을 업데이트 시켜 각 노드의 최적의 weight을 찾는 방식으로 작동 된다. ANN(Artificial Neural Network) ANN 문제점 - 학습과정에서 최적의 파라미터를 찾기 어렵다 sigmoid 함수의 문제점 때문 (local minima를 global minimum으로 인식) - Overfitting 문제 - 학습 시간이 너무 느리다 은닉층 증가될 수록 연산량 급격히 증가 ANN 문제 개선 - overfitting -> initialize p..
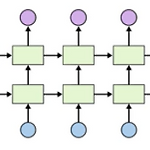
모두를 위한 딥러닝 ML lab12-4: RNN with long sequences: Stacked RNN + Softmax layer 강의 정리&코드 전체 코드: https://github.com/hunkim/DeepLearningZeroToAll/blob/master/lab-12-3-char-seq-softmax-only.py RNN의 핵심 - Wide & Deep 1. MultiRNNCell MultiRNNCell을 이용해서 cell을 쌓아보자 원하는 만큼 많이 쌓을 수 있음 cell = rnn.MultiRNNCell([cell] * 2 , state_is_tuple=True) # [cell]* n에서 n: 몇 개 쌓을 것인가 * states 변수는 층마다 하나의 텐서가 들어 있는 튜플이다. (셀의..
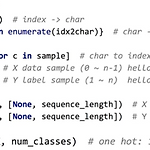
모두를 위한 딥러닝 ML lab12-3: Long Sequence RNN 강의 정리&코드 전체 코드: https://github.com/hunkim/DeepLearningZeroToAll/blob/master/lab-12-2-char-seq-rnn.py 1. data creation # Data creation idx2char = ['h', 'i', 'e', 'l', 'o'] # Teach hello: hihell -> ihello x_data = [[0, 1, 0, 2, 3, 3]] # hihell x_one_hot = [[[1, 0, 0, 0, 0], # h 0 [0, 1, 0, 0, 0], # i 1 [1, 0, 0, 0, 0], # h 0 [0, 0, 1, 0, 0], # e 2 [0, 0, 0,..
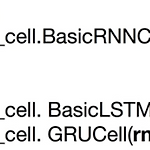
모두를 위한 딥러닝 ML lab12-2: RNN - Hi Hello Training 강의 정리&코드 전체 코드: https://github.com/hunkim/DeepLearningZeroToAll/blob/master/lab-12-1-hello-rnn.py 1. RNN model 적합한 것 골라 쓰기 2. Data creation idx2char = ['h', 'i', 'e', 'l', 'o'] # Teach hello: hihell -> ihello x_data = [[0, 1, 0, 2, 3, 3]] # hihell x_one_hot = [[[1, 0, 0, 0, 0], # h 0 [0, 1, 0, 0, 0], # i 1 [1, 0, 0, 0, 0], # h 0 [0, 0, 1, 0, 0], # e..
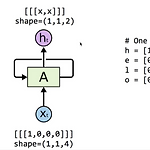
모두를 위한 딥러닝 ML lab12-1: RNN - Basics 강의 정리&코드 전체 코드: https://github.com/hunkim/DeepLearningZeroToAll/blob/master/lab-12-0-rnn_basics.ipynb 1. 데이터 # One hot encoding h = [1, 0, 0, 0] e = [0, 1, 0, 0] l = [0, 0, 1, 0] o = [0, 0, 0, 1] # input dimension -> 4 (0,0,0,0) 입력 데이터의 dimension이 뭐든 상관없이 출력의 dimension은 우리가 설정한 hidden_size를 따름 2. cell 만들기 with tf.variable_scope('one_cell') as scope: hidden_siz..